- April 26, 2023
- Posted by: admin
- Category: BitCoin, Blockchain, Cryptocurrency, Investments
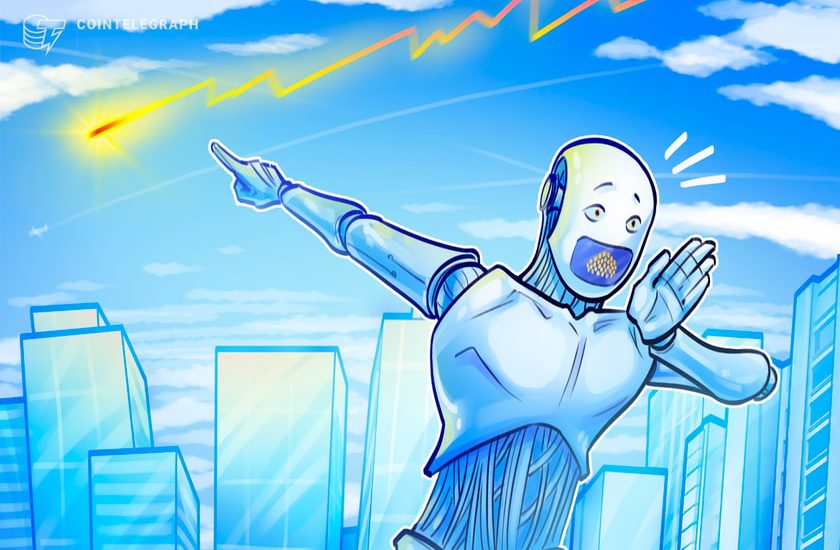
This article explores whether AI can prevent the next financial crisis by improving risk management and identifying potential threats.
What are the ethical considerations in the use of AI for financial risk management?
Financial institutions using AI for risk management must ensure diverse and impartial data, transparency in decision-making, responsible outcomes, data security and privacy, human supervision and accountability for decisions, consider AI’s impact on employment, and use the technology ethically.
The accuracy of AI algorithms depends on the data used to train them. Financial institutions must therefore make sure that the data they employ is diverse, impartial and representative of all societal groups.
Financial institutions must be open and explain their decision-making processes when using AI for risk management. They must also take responsibility for any unforeseen outcomes that may result from the use of AI.
Large volumes of personal data are needed for AI, which prompts questions regarding data security and privacy. Financial institutions must make sure that they are using data in a secure and ethical manner and that they have the necessary security measures in place to prevent data breaches.
AI is a tool that can assist with decision-making, but in the end, decisions must be made by humans. Financial institutions must therefore make sure that choices made using AI are subject to human supervision and accountability.
The rising use of AI in managing financial risks could result in job losses and changes to the nature of work. Financial institutions must be aware of how AI may affect employment and make sure they are using the technology ethically.
What are some potential benefits and limitations of using AI in financial risk management and prevention?
AI has many potential benefits in financial risk management, including improved accuracy, real-time monitoring, improved productivity, cost-effectiveness and predictive analytics. However, there are also limitations, such as lack of transparency, data quality issues, potential biases, over-reliance on AI and cybersecurity risks that must be considered before implementing AI-powered solutions in financial institutions.
Using AI in financial risk management and prevention has many potential benefits, including:
- Improved accuracy: AI can help identify potential risks more accurately and quickly than traditional methods, which can improve the effectiveness of risk management and prevention efforts.
- Real-time monitoring: AI can track client behavior and transactional data in real-time, enabling financial institutions to spot fraud and other threats as they develop.
- Improved productivity: AI-powered risk management solutions can automate a variety of processes, giving analysts more time to concentrate on higher-level work.
- Cost-effective: AI can assist financial organizations in lowering the expenses associated with risk management by automating tasks and decreasing the need for manual review.
- Predictive analytics: By using past data to forecast potential risks and trends, predictive analytics enables financial organizations to proactively manage potential risks.
However, there are also some limitations to using AI in financial risk management and prevention, including:
- Lack of transparency: It can be challenging to comprehend AI-powered systems, which makes it challenging for financial institutions to explain how choices are made.
- Data quality: For AI to be effective, high-quality data is essential, yet low-quality data might result in incorrect predictions and judgements.
- Bias: AI can be biased if the data used to train the system is biased or if the algorithms themselves are biased.
- Over-reliance on AI: Financial institutions may become too reliant on AI-powered systems, which can lead to complacency and a lack of human oversight.
Cybersecurity risks: AI-powered systems may be vulnerable to cyberattacks, which can compromise the security of sensitive financial data.
What are some examples of AI-powered fraud detection systems for financial institutions?
A few examples of AI-powered fraud detection systems that financial institutions can use to protect their customers from fraudulent activities include FICO Falcon Fraud Manager, Feedzai, IBM Safer Payments, NICE Actimize and Featurespace ARIC Fraud Hub.
FICO Falcon Fraud Manager
FICO Falcon Fraud Manager is a fraud detection and prevention system that analyzes client transactions in real-time, using artificial intelligence and machine learning techniques. Suspected fraud can be identified by the system, which can also notify the bank’s fraud management team.
Feedzai
Feedzai is a solution for detecting probable fraud that analyzes client transactions using machine learning techniques. It can analyze customer behavior and identify patterns that may indicate fraud. For example, if a customer suddenly starts making large purchases or purchases in unusual locations, Feedzai can flag this as potentially fraudulent activity.
IBM Safer Payments
IBM Safer Payments is a system for detecting and preventing payment fraud that employs artificial intelligence and machine learning techniques. Based on patterns of behavior, transaction history and other variables, the system can spot possible fraud.
NICE Actimize
NICE Actimize is a financial crime detection system that analyzes customer data and spots probable fraudulent activity using artificial intelligence and machine learning techniques. It provides solutions for Know Your Customer (KYC) and customer due diligence, which help financial institutions verify the identity of their customers and comply with regulatory requirements.
Featurespace ARIC Fraud Hub
Featurespace ARIC Fraud Hub is a real-time fraud detection system that scans client transactions for possible fraud, using machine learning algorithms. It can detect and prevent fraud in real-time, allowing financial institutions to respond quickly and prevent further losses.
How can AI help develop early warning systems for potential risks?
By analyzing massive volumes of data in real-time and giving decision-makers useful insights, AI can assist in the development of early warning systems that can identify possible problems in financial markets.
Here are the steps that AI can take to help develop early warning systems:
Data collection
AI systems are capable of gathering information from a range of sources, such as financial accounts, news articles and social media feeds.
Data preprocessing
The obtained data needs to be preprocessed in order to weed out any unnecessary information and put it in a format that can be used for analysis.
Feature selection
The next step is to choose the features that are most likely to be indicative of possible risks in the preprocessed data. Variables like cryptocurrency prices, interest rates, credit ratings and economic indicators may be included in this.
Machine learning
Once the pertinent features have been chosen, models that can anticipate possible risks can be trained using machine learning methods. These models can be trained using historical data to spot trends that could portend the beginning of crises, such as systemic risk, credit crunch, bankruptcy, debt crisis or a stock market catastrophe.
Early warning system
Early warning systems can be created using machine learning models once they have been trained to advise stakeholders of potential threats. These technologies can also be used to assess the risk’s seriousness and offer potential mitigation measures.
For instance, by examining historical price data, an AI-based early warning system could spot a pattern where a certain cryptocurrency’s price is declining unusually quickly. This might be a forerunner to a systemic risk, which could lead to a credit crunch or a crypto market collapse. Market participants might be informed of this tendency by the system, allowing them to take preventative measures to reduce the risk.
Related: What is crypto contagion, and how does it affect the market?
What role can AI play in preventing the next financial crisis?
By analyzing vast amounts of data in real-time, AI can identify potential risks and provide early warnings to enable proactive measures. However, addressing challenges such as transparency and interpretability is vital to ensuring the responsible and effective use of financial services.
AI has the potential to play a significant role in preventing the next financial crisis by improving risk management and enhancing decision-making processes. To identify key hazards and provide early warnings of prospective financial crises, AI can examine complicated correlations between various economic indicators, financial markets and global events by processing enormous volumes of data in real-time. This can assist financial firms and regulators in taking preventive steps to reduce risks and avert disasters.
AI can also be used to create predictive models that can predict market patterns and spot potential risks before they occur. This can assist financial institutions in managing their risk exposure appropriately and adjusting their investment strategy. AI can also be used to better detect fraud and stop financial crimes, which can be a major cause of instability in the financial system.
Related: What are artificial intelligence (AI) crypto coins, and how do they work?
Predictive models are statistical models or machine learning algorithms that are used to analyze historical data and make predictions about future events or behaviors. For instance, suppose that a bank wants to identify the clients who are most likely to default on their loans.
The bank can train a machine learning system to find trends connected to defaults using past data on customer credit ratings, income levels, job status and other pertinent criteria. The algorithm can then be used to create a predictive model that gives each client a risk score and predicts how likely they are to default.
With the use of this prediction model, the bank may focus on clients who are most at risk of default and allocate its resources accordingly. It can present them with other payment options or collaborate with them to solve the underlying problems that might be causing their financial problems. By using a predictive model, the bank can proactively manage its loan portfolio and minimize losses due to defaults.
The use of AI in financial services is not without difficulties, though. One of the key issues is that AI models lack transparency and interpretability, which can make it challenging to comprehend the justification for judgements made by AI. This can be solved by creating transparent explainable AI (XAI) models that permit human monitoring and involvement.
XAI refers to a class of artificial intelligence techniques and methods that are designed to produce human-understandable explanations for the decisions and actions taken by AI systems. This can be particularly crucial in fields like banking, healthcare or criminal justice where judgements made by AI systems may have far-reaching effects. Using XAI can assist in improving the effectiveness and dependability of AI systems as well as their openness, accountability and fairness.
Is AI recession-proof?
While AI is not recession-proof, it can help companies recover from a recession by improving business efficiency, identifying new opportunities and preventing future financial instability.
Even if artificial intelligence (AI) has the potential to enhance company productivity and decision-making, it is not recession-proof. It is because the performance of AI models during a financial or economic crisis depends on the data on which they were trained.
AI may be unable to make accurate predictions or insights if the available data is outdated, biased or insufficient. Moreover, AI demands a substantial investment, and during a recession, businesses might be reluctant to make such expenditures.
AI, on the other hand, can support business recovery in a number of ways. For instance, it can assist businesses in cost-cutting and operational optimization, allowing them to weather the economic storm.
AI can also help businesses in locating new markets and commercial prospects, which may result in the creation of new revenue streams. Additionally, by offering real-time monitoring and early warning systems, AI can enhance risk management and avert future financial instability.
Furthermore, AI has the potential to contribute to future economic development by stimulating innovation and creating new jobs in the future. Robotics and automation systems that use AI can boost output and efficiency, which boosts the economy.